About the basis of IEQ
Several factors influence the Indoor Environmental Quality (IEQ). They consist of thermal, acoustic, optic, and atmospheric environment and indoor air quality (IAQ). The definitions, requirements, and standards for these factors are described below.
1. Thermal comfort
Two models can be used to analyze thermal comfort: the static predicted mean vote (PMV) / the predicted percentage of dissatisfied (PPD) developed by Fanger and the adaptive Model.
1.1 PMV/PPD-Modell
Fanger [1] first introduced the PMV/PPD model in the 1970s to evaluate thermal comfort. The following factors largely define the subjective perception of thermal comfort [2]:
- Air temperature indoors [°C]
- Mean radiant temperature [°C]
- Relative air humidity [%]
- Relative air speed [m/s]
- Clothing [clo]
- Physical activity [met]
The first four parameters are physical factors of the environment and the rest define the ability of the body to adapt itself to the environment to hold the thermal balance [3]. With these factors, the PMV index can be calculated and used to predict the thermal sensitivity of the body. [2]
PMV
The PMV model by Fanger [1] is a tool for general or whole-body thermal comfort prediction. It was based on laboratory and environmental chamber research in the second half of the 1960s. Because of its structure based on balance equations (see below) is the PMV/PPD model often described as a static model. [4]
PMV = (heat flow of the body) – (required heat flow for optimal comfort)
The energy balance equation by Fanger is as follows:
$$M – W = (S_D + E_{SW}) + (R_D + R_W) + (C + R)$$
Definition of factors:
- Net energy production:
- M: Body’s energy production (heat) in W/m2
- W: Usable mechanical power of the muscles (exergy) in W/m2
- Sweating/Evaporation
- SD: Heat loss caused by water vapor diffusion on the skin (insensible) in W/m2
- A subconscious reaction of the body (also: perspiratio insensibilis) $$S_D = 0.00305 \cdot (5733 – 6.99 \cdot (M-W) – p_D)$$
- pD: Water vapor partial pressure in Pa
- ESW: Heat loss due to evaporation of sweat on the skin (sensible) in W/m2
- A controlled variable representing the regulation of the skin temperature (also: perspiratio sensibilis)
- For M > 58.15 W/m²: $$E_{SW} = 0.42 \cdot ((M – W) – 58.15)$$
- SD: Heat loss caused by water vapor diffusion on the skin (insensible) in W/m2
- Breathing:
- RD: Heat loss of dry breathing air in W/m2
- Depends on the indoor air temperature ϑa [°C] $$R_D = 0.0014 \cdot M \cdot (34 – \vartheta_a)$$
- RW: Heat loss of humid breathing air
- Depends on the water vapor partial pressure pD [Pa] $$R_W = 0.000017 \cdot M \cdot (5867 – p_D)$$
- RD: Heat loss of dry breathing air in W/m2
- Convection and solar radiation:
- C: Heat loss due to convection on the surface area of a clothed body in W/m2
- Depending on the indoor air temperature ϑa [°C], the surface area temperature of a clothed body ϑcl [°C], the convective heat transfer coefficient hc [W/m²·K], the ratio of the clothed and not clothed body surface area fcl [-]. $$C = f_{cl} \cdot h_c \cdot (\vartheta_{cl} – \vartheta_a)$$
- Convective heat transfer coefficient hc depends on the relative air speed vrel [m/s]
- Forced convection $$h_c = 12.1 \cdot \sqrt{v_{rel}}$$
- Free convection $$h_c = 2.4 \cdot (\vartheta_{cl} – \vartheta_a)^{0.25}$$
- Note, that the bigger index of both is used
- R: Heat loss due to solar radiation on the surface area of a clothed body in W/m2
- Depends on the ratio of the clothed and not clothed body surface area fcl [-], the temperature of the surface area of a clothed body ϑcl [°C], mean solar radiation of the surrounding areas ϑr [°C].$$R = 3.96 \cdot 10^{-8} \cdot f_{cl} \cdot [(\vartheta_{cl} + 273)^4 – (\overline{\vartheta_r} + 273)^4]$$
- For fcl is Icl the insulation value of clothing $$f_{cl} = 1.00 + 1.29 \cdot I_{cl} \qquad (I_{cl} \leq 0.078 \frac{m^2 \cdot K}{W})$$
- $$f_{cl} = 1.05 + 0.645 \cdot I_{cl} \qquad (I_{cl} > 0.078 \frac{m^2 \cdot K}{W})$$
- C: Heat loss due to convection on the surface area of a clothed body in W/m2
After disassembling of the Fanger equation, the PMV index can be calculated with this formula: $$L = M – W – (S_D + E_{SW}) – (R_D + R_W) – (C + R)$$
L [W/m²] for the thermal Load describes the heat transport from the skin surface through clothing and to the clothing surface (or also through breathing), considering the general energy balance equation. The PMV index is then calculated using empirical correlation: $$PMV = (0.303 \cdot e^{-0.036 \cdot M} + 0.028) \cdot L$$
The PMV is an index that predicts the average value for the indoor climate assessment by a large group of people. It uses the following 7-point climate assessment scale; see Table 1 [2].
Index | Assessment |
+3 | hot |
+2 | warm |
+1 | slightly warm |
0 | neutral |
-1 | slightly cool |
-2 | cool |
-3 | cold |
Which factors does the Fanger formula include?
Physical activity [met]
The physical activity or the level of activity M describes the energy a body generates during the exchange of chemical energy into mechanical and thermal energy. The activity level is important for determining thermal comfort because, in a (very) warm environment, the extra body heat that’s being produced during the muscle activity increases the heat load.
The energy expenditure is converted from W/m2 into a metabolic unit met: $$1 \: met = 58 \frac{W}{m^2}$$
70 W/m2 or1,2 met corresponds to the average activity for “Seated Activities (office, apartment, school, laboratory). Additional energy expenditures can be found in ISO 8996.
The mechanical efficiency of a muscle, W (also usable work), is low. In most work activities, this is generally so low that it is considered to be zero and is only taken into account from 4.0 to 4.5 METs (Metabolic Equivalent of Task).
Clothing [clo]
The thermal insulation value for clothing is measured in unit clo: $$1 \: clo = 0.155 \frac{m^2K}{W}$$
For a typical clothing combination of “underwear, shirt, pants, socks, shoes,” an insulation value of 0.70 clo can be assumed. The additional thermal insulation in the area of seat contact surfaces should also be considered (e.g., 0.01 clo for a wooden stool and 0.1 clo for a typical office chair). These values can be added to the values of typical clothing insulation. The corresponding insulation values for clothing and seat contact surfaces can be found in DIN EN ISO 7730 and DIN EN ISO 9920.
PPD
The Predicted Percentage of Dissatisfied (PPD) provides information about thermal comfort or discomfort by predicting the percentage of people who are likely to find a particular environment too warm or too cold. The PPD can be calculated from the Predicted Mean Vote (PMV) [2]. It is the result of numerous surveys conducted with test subjects, and the mathematical relationship between PMV and PPD is based on data from over 1,000 individuals. It should be noted that the comfort equation derived by Fanger applies to moderate climate zones [1].
$$PPD = 100 – 95 \cdot e^{-0.03353 \cdot PMV^4 – 0.2179 \cdot PMV^2}$$
ISO 7730 [2] recommends a maximum PPD value of around 10% in buildings. Figure 1 shows the relationship between PMV and PPD in various studies, where data was collected in naturally ventilated buildings and climate chamber environments in East Asia, South America, and Germany. The discrepancies in the results of these studies could be because thermal neutrality is not always the ideal situation for occupants, as some people prefer non-neutral thermal sensations (e.g., slightly warm or slightly cool). These sensations may even be asymmetrically distributed around thermal neutrality and can be influenced by the seasons. Moreover, the discrepancy could be attributed to the variability between the studied populations, the sample size, and the definition of discomfort [4].

The PMV model should be used globally for all building types. However, it was developed based on laboratory studies and does not take into account the building type or the influence of environmental psychology. Thermal comfort is not just the sum of technical and physiological aspects but also includes social and psychological conditions [4]. Therefore, the limitations of the PMV/PPD model must be considered when applying it.
According to de Dear and Brager [5], the PMV model does not apply to naturally ventilated buildings, as it only partially accounts for thermal adaptation to indoor climates. These reasons led to the development of adaptive thermal comfort models.
1.2 Adaptive model
The hypothesis of adaptive thermal comfort suggests that environmental factors and thermal history influence the thermal expectations and preferences of occupants. People living in warmer climates prefer higher indoor temperatures than those living in colder climates, contrary to the comfort criteria based on the PMV model [6]. This adaptation can be physiological (short-term acclimation or long-term acclimatization), psychological (expectations), and/or behavioral (opening windows, choosing clothing, etc.).
The study by de Dear [7] found that in naturally ventilated buildings, the indoor temperature perceived as most comfortable was significantly higher in warmer climate regions and lower in colder ones. As described in DIN EN 16798-1 [8], the adaptive method applies to office buildings without mechanical cooling and other similar buildings primarily intended for use by people engaged in mostly sedentary activities, that provide easy access to operable windows, and where occupants can freely adjust their clothing to suit indoor and/or outdoor thermal conditions.
DIN EN 16798-1 [8] defines four categories of indoor climate quality based on expectations, as shown in Table 2. These categories correspond to the level of potential expectations of building occupants. An average level of expectations would be considered “medium” (PPD < 10%). For occupants with special needs (children, elderly, people with disabilities, etc.), a higher level of expectations can be selected. A lower level of expectations does not pose health risks but may reduce comfort.
Category | Level of expectations | PPD [%] | PMV |
I | high | < 6 | -0,2 < PMV < +0,2 |
II | average | < 10 | -0,5 < PMV < +0,5 |
III | moderate | < 15 | -0,7 < PMV < +0,7 |
IV | low | < 25 | -1,0 < PMV < +1,0 |
Figure 2 shows the recommended ranges of operative indoor temperature for buildings without artificial cooling systems, depending on the moving average of the outdoor temperature as defined below.

The moving average of the outdoor temperature is calculated according to this formula [8]: $$\theta_\mathrm{rm}=(1-\alpha)\cdot (\theta_\mathrm{ed-1}+\alpha \cdot \theta_\mathrm{ed-2}+\alpha^\mathrm{2}\theta_\mathrm{ed-3}+…+\alpha^\mathrm{i}\theta_\mathrm{ed-i})$$
With the following factors:
- θrm: the moving average of the outdoor air temperature on the day of the observation [°C]
- θed−i: daily average of the outdoor air temperature for the previous day i [°C] (advised value in DIN EN 16798-1: i = 7)
- α: a constant between 0 and 1 (advised value in DIN EN 16798-1: α = 0,8)
The constant α is between 0,6 and 0,9 depending on each case. The effects are illustrated below in the Figure 3.
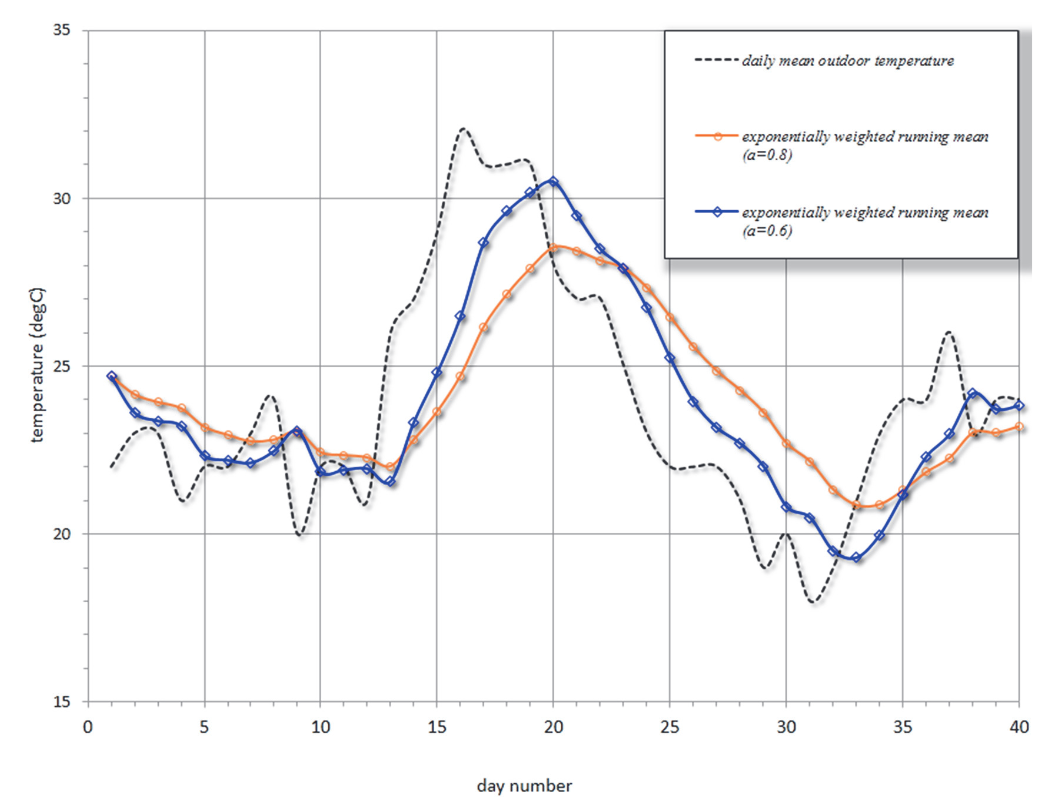
The equation for the operative indoor temperature from Figure 2 is represented in the following table 3.
Category | Position | Equation |
I | upper bound | $$\theta_\mathrm{o}=0.33 \cdot \theta_\mathrm{rm}+18.8+2$$ |
I | in the middle | $$\theta_\mathrm{c}=0.33 \cdot \theta_\mathrm{rm}+18.8$$ |
I | lower bound | $$\theta_\mathrm{o}=0.33 \cdot \theta_\mathrm{rm}+18.8-3$$ |
II | upper bound | $$\theta_\mathrm{o}=0.33 \cdot \theta_\mathrm{rm}+18.8+3$$ |
II | lower bound | $$\theta_\mathrm{o}=0.33 \cdot \theta_\mathrm{rm}+18.8-4$$ |
III | upper bound | $$\theta_\mathrm{o}=0.33 \cdot \theta_\mathrm{rm}+18.8+4$$ |
III | lower bound | $$\theta_\mathrm{o}=0.33 \cdot \theta_\mathrm{rm}+18.8-5$$ |
The adaptive comfort model takes into account not only behavioral adaptations (e.g., changes in clothing) but also the temporal development of the outdoor climate over the past few days. This makes it a useful complement to the Fanger model, although it also involves greater effort.
1.3 Local thermal comfort
The PMV/PPD model and the adaptive model describe the sensation of heat and cold for the entire body. However, thermal discomfort can also be caused by unwanted cooling (or heating) of a specific body part, referred to as local thermal discomfort. The most common cause of local thermal discomfort is draught. It can also result from significant temperature differences between the head and feet, excessively warm or cold floors, or significant asymmetry [2].
Local thermal discomfort is primarily experienced by individuals engaged in light sedentary activities. This group tends to have a nearly neutral thermal sensation for the entire body. At higher activity levels, the thermal sensation of individuals is generally less pronounced, resulting in a lower risk of local thermal discomfort [2].
The advised maximal airspeed to avoid draught from DIN EN 16798-1 is given in the table below [8]:
Category | Draught rate | Maximal airspeed |
[%] | Winter [m/s] | Summer [m/s] | |
I | 10 | 0.10 | 0.12 |
II | 20 | 0.16 | 0.19 |
III | 30 | 0.21 | 0.24 |
The values in Table 4 apply to ambient conditions with an activity level of 1.2 met, a turbulence level of 40%, and a temperature that equals to operative temperature. Although DIN EN 16798-1 does not recommend high air velocity, increased air speed can be used to compensate for the sensation of heat caused by elevated temperatures [8]. The benefit of increased air speed depends on clothing, physical activity, and the difference between the surface temperature of clothing/skin and the air temperature [2].
Figure 4 shows the air ызуув required for a typical summer office environment (0.5 clo and 1.2 met) to achieve comfort. For the application of Figure 4, occupants need to have a certain degree of personal control over the air speed. [4].

1.4 Additional thermal comfort models
Combination of PMV models and adaptive components
- aPMV – Black Box approach and expansion with adaptive coefficients [10].
- Coefficient λ stands for all the influencing factors that aren’t included in the Fanger equation (culture, climate, behavior)
- Research needs to be done to adapt the corrections; they are not universally applicable. $$aPMV = \frac{PMV}{1 + \lambda \cdot PMV}$$
- PMVe – Focus on one component of the adaptive model [11].
- Expected value e depends on the outdoor climate and lies between 0,5 ~ 1,0 $$PMV_e = PMV \cdot e$$
Thermophysiological models
- DTS – Dynamic Thermal Sensation Model [12]
- This model is based on the physiological principles of thermal comfort.
- Passive Factors:
- Heat transfer processes in the body (blood circulation, heat generation, conduction, and storage) and at the body surface (convection, longwave radiation, solar radiation, evaporation of skin moisture, diffusion, and accumulation).
- Uneven heat and evaporation resistance of the entire garment and changes in the effective body radiation surface depending on body posture.
- Active Factors:
- The response of the human thermoregulation system.
- Zhang’s model / Berkeley model – local thermal comfort focused on an inhomogeneous environment [13-15]
- 19 individual local body parts $$Local \; Sensation \; S_l = f(T_{skin,i}, \; \frac{dT_{skin,i}}{dt}, \; \overline{T}_{skin}, \; \frac{dT_{core}}{dt})$$
- $$Local \; Comfort = Logistic \; Function(S_l + offset) + maxcomfort$$
- For each body part, the sensations of warmth and cold are weighted differently, with strong localized sensations dominating the overall perception.
- When all sensations are approximately neutral, the overall feeling is close to the average of all body sensations.
- BSM – Balance Comfort Model: Local Comfort Model with a focus on inhomogeneous environments [16]
- Heat balance for individual body parts (10 segments) and the entire body.
- Integration of heat conduction through contact problems into the energy balances as the dominant heat exchange process for contacting body areas.
- Individualization of the comfort model is possible by using individual energy balance terms or parameters (e.g., metabolic rate, local clothing insulation).
- Implementation of the comfort models in MODELICA/Dymola.
- JOS3 – Joint System Thermoregulation Model [82]
- 85 points (17 body parts), based on [80, 81]
- Taking into account personal characteristics (BMI, age, etc.) in unsteady and inhomogeneous thermal environments.
- Integration with CFD simulation and Python.
- ATHB – Adaptive Thermal Heat Balance Model [17]
- Physiological (derived from the basal metabolic rate)
- Behavioral (degree of clothing) $$clothing \; adjustments = \beta_0 + \beta_1 \cdot T_{rm} + \beta_2 \cdot MET + \beta_3 \cdot Building \; Type$$
- Physiological correction (Comparison of the Thermal Load L and Actual Thermal Sensation ATS)
Personalised thermal comfort models
Instead of the average feedback calculated from the data of a group of people, a personalized thermal comfort model is based solely on the thermal data of an individual. Martins et al. [18] published a review article on personalized models for thermal comfort. For more details, see https://doi.org/10.1016/j.buildenv.2021.108502.
2. Acoustic comfort
Any sound perceived as undesirable by an occupant can be considered noise. A poor acoustic environment in offices makes users unable to concentrate on their work [19]. In addition to performance, acoustic quality can also affect the comfort and health of occupants [20]. A study led by the WHO quantified the effects of environmental noise on the health of residents, highlighting the negative impacts of noise on sleep disturbances, cardiovascular diseases, and tinnitus [21]. Navai and Veitch [22] define acoustic comfort as “the state of satisfaction with the acoustic environment.”
Frontczak [23], however, argues that the term “acoustic comfort” is not widely used and that providing a good acoustic environment is mainly focused on avoiding discomfort (annoyance). The quality of the sound environment depends on numerous physical parameters, both the physical properties of the sound itself and the physical characteristics of the space. The acoustic environment is influenced by physical room properties such as sound insulation, absorption, and reverberation time [24]. The most commonly measured acoustic parameters for assessing acoustic quality are reverberation time and the A-weighted sound pressure level/noise level in dB(A), as these two parameters are widely referenced in standards/guidelines [20].
For offices, Keighley [25] suggested an acceptable upper limit of 68 dB(A), Warnock [26] recommended 48 dB(A), and Keighley et al. [27] proposed 46 dB(A). However, since these studies were conducted earlier and the nature of the work is unknown, it is difficult to assess the applicability of the results [22].
In DIN EN 16798-1, the requirements for system-related indoor noise levels for certain rooms and buildings are specified in Table 5, where only the requirements for offices and residential buildings are listed here. The values in Table 5 refer to the noise caused by technical building equipment systems within the room under consideration [8].
Building | Room type | Equivalent continuous sound level LAeq,nT [dB(A)] – Category I | Equivalent continuous sound level LAeq,nT [dB(A)] – Category II | Equivalent continuous sound level LAeq,nT [dB(A)] – Category III |
Residential buildings | Living room | ≤ 30 | ≤ 35 | ≤ 40 |
Residential buidlings | Bedroom | ≤ 25 | ≤ 30 | ≤ 35 |
Office | Small offices | ≤ 30 | ≤ 35 | ≤ 40 |
Office | Office landscapes | ≤ 35 | ≤ 40 | ≤ 45 |
Office | Conference rooms | ≤ 30 | ≤ 35 | ≤ 40 |
3. Visual Comfort
Light influences people’s mood, emotions, and mental alertness. It can also support and adjust the circadian rhythm, as well as affect an individual’s physiological and psychological state. Different light intensities at various times of the day and year, along with varying color temperatures, can enhance people’s well-being [28].
Inadequate lighting negatively impacts users’ attention and work performance, as their eyes become strained while in the office [19]. DIN EN 12464-1 [28] provides detailed requirements for illuminance levels, as outlined in Table 6.
Ref. Nr. | Building and room type – area of the visual task/activity | Em,r [lx] – Required Value | Em,u [lx] – recommended Value | Required light in the immediate surrounding area [lx] |
6.26.2 | Offices – writing, typing, writing, data processing | 500 | 1000 | 300 |
6.26.3 | Offices – technical drawing | 750 | 1500 | 500 |
6.26.5.1 | Offices – Conference and meeting rooms | 500 | 1000 | 300 |
6.33.2 | Libraries – reading area | 500 | 750 | 300 |
6.35.2 | Educational institutions – Kindergarten | 300a | 500a | 200 |
6.36.1 | Educational institutions – classroom – general activities in primary schools | 300b | 750b | 200 |
6.36.2 | Educational institutions – classroom – general activities in schools | 500b | 1000b | 300 |
6.36.3.1 | Educational institutions – Auditoriums, lecture halls | 500b | 750b | 300 |
- High luminance levels should be avoided in the viewing direction from below by using diffuse covers.
- The light intensity should be controllable
In addition to the required illuminance levels, additional qualitative and quantitative requirements must be met for good lighting. The lighting requirements are determined by fulfilling three fundamental human needs [28]:
- Visual comfort, in which employees feel comfortable, indirectly contributing to higher productivity and quality of work.
- Visual performance enables employees to manage their visual tasks even under challenging conditions and for extended periods.
- Safety.
In addition to light intensity, the following parameters also play an important role in visual comfort [28]:
- Light distribution
- Direction of light, spatial illumination
- Light variability (brightness and color of light)
- Color rendering and color of the light
- Glare
- Flicker
Large local differences in illuminance in the vicinity of a visual task or activity can lead to visual strain and discomfort. In addition to illuminating areas for visual tasks and activities, the space used by individuals should also have enough light. This light is necessary to highlight objects, make textures visible, and enhance the appearance of people in the space. The required lighting in the immediate surrounding area for typical buildings and rooms can also be found in Table 6 [28].
4. Barometric Comfort
Several studies have shown that barometric conditions can directly influence well-being or illness:
People with arthritis often believe that weather conditions (air pressure, temperature, etc.) affect the severity of their pain. Air pressure, relative humidity, and sunshine have a significant influence on joint pain [29]. Surveys among people with rheumatic diseases have shown that one-third to two-thirds believe their symptoms are weather-dependent [30]. McAlindon’s study [31] also demonstrated that increased air pressure correlates with intensified osteoarthritis pain. A study conducted in Nepal in 2017 [32] found that living at high altitudes not only increases the prevalence of migraines but also the severity of their symptoms.
However, it is important to note that establishing a consistent causal relationship between joint pain and air pressure is challenging. In the medical community, this belief is generally regarded as a psychological misperception [31].
In contrast to the direct effects previously described, it is more likely that different barometric environments have an indirect effect on thermal comfort, for example. This occurs by influencing physiological conditions such as metabolic rate and heart rate, etc. [75-79]. The PMVaps model, which considers the effects of barometric pressure on thermal comfort based on the PMV/PPD model, was developed by Ruivo et al. [79]. The correlation between barometric pressure and metabolic rate was confirmed by Liu et al. [76] through experiments in high-altitude regions. The effects of air pressure on inter-physiological parameters such as oxygen saturation and heart rate have also been reported by Hu et al. [75].
5. Indoor air quality (IAQ)
In recent decades, indoor air quality (IAQ) has received increasing attention to improve the comfort and health of building occupants. It is estimated that people spend about 90% of their time in private or public spaces, such as homes, schools, and offices. Consequently, IAQ often has a significant impact on health and quality of life [33]. Since the outbreak of the COVID-19 pandemic, IAQ, which is typically estimated through CO2 measurements, has gained particular attention and serves as a proxy for the air exchange rate [34, 35].
Indoor air pollution has substantial effects on people’s quality of life due to the impact of harmful chemicals such as carbon monoxide (CO), ozone (O3), volatile organic compounds (VOCs), particulate matter (PM), and other toxic substances. It can be up to ten times worse than outdoor air pollution [36]. Poor IAQ can particularly harm vulnerable groups, such as children, adolescents, the elderly, and individuals with chronic respiratory or cardiovascular conditions [37].
Additionally, poor IAQ can also affect productivity. High levels of air pollution, even if not perceived as significant by the occupants, can reduce productivity. To 8improve IAQ, it is often more energy-efficient to eliminate sources of air pollution than to increase air supply through, for example, HVAC systems [38].
A widely used indicator for assessing air quality is the Air Quality Index (AQI) defined by the U.S. Environmental Protection Agency (EPA) [44], which can take values between 0 and 500. A lower value indicates better air quality. The specific intervals are listed in Table 7 below.
AQI | Definition | Color |
0 ~ 50 | Good | Green |
51 ~ 100 | Moderate | Yellow |
101 ~ 150 | Unhealthy for Sensitive Groups | Orange |
151 ~ 200 | Unhealthy | Red |
201 ~ 300 | Very Unhealthy | Purple |
301 ~ 500 | Hazardous | Brown |
The EPA’s AQI analyses air pollutants, including ozone (O3), PM2.5, PM10, CO, and SO2. It does not differentiate between typical indoor and outdoor air pollutants. Table 8 lists the sensitive populations that are affected when the aforementioned air pollutants exceed the specified levels (AQI over 100) [44].
When the pollutant has an AQI of over 100 | Sensitive population groups |
Ozon (O3) | The most vulnerable groups are people with lung diseases, children, older adults, and individuals who spend a lot of time outdoors. |
PM2.5 | The most vulnerable groups are people with heart or lung diseases, older adults, and children. |
PM10 | The most vulnerable groups are individuals with heart or lung diseases, older adults, and children. |
CO | The population group with heart diseases is particularly affected by such hazards. |
SO2 | The population group with heart disease is particularly affected by such hazards. |
Some manufacturers of gas sensors have developed algorithms that allow the conversion of measurements into a range of 0 to 500, ensuring compatibility with the AQI. This way, air quality can be displayed, as seen with the VOC sensor SGP40 from Sensirion [45]. However, there are two aspects to consider:
- The original AQI from the EPA only includes the air pollutants listed in Table 8 and does not account for gases typically measured indoors, such as VOCs and CO2.
- The index range of 0 to 500 in the gas index algorithms developed by manufacturers may deviate from the original definition of the AQI set by the EPA, as shown in Figure 5.

This section focuses on the relationship between VOCs, CO2, and indoor air quality (IAQ).
Volatile Organic Compounds VOC
VOCs (Volatile Organic Compounds) are defined as organic compounds that have a vapor pressure of 0.01 kilopascals or more at 293.15 Kelvin or exhibit corresponding volatility under the conditions of their use, such as propylene glycol and glycol ethers, benzene, and formaldehyde [39]. VOCs are commonly found in consumer goods such as furniture, sealants, and paints. They strongly react with the epithelial lining of the respiratory tract and mucous membranes and are associated with lung diseases such as asthma [40].
According to the EPA, VOC concentrations indoors in the United States are two to five times higher than concentrations outdoors. VOCs such as propylene glycol, glycol ethers, benzene, and formaldehyde strongly react with the epithelial cells of the respiratory tract and mucous membranes [41]. This means that VOCs can trigger inflammation of the airways [40].
Although ventilation or air purifiers can reduce VOC concentrations, they do not eliminate the sources of VOCs, as consumer goods like furniture and paints can continue to emit VOCs for months to years [40, 42]. Therefore, it is essential to continuously monitor VOC concentrations in indoor air and minimize VOC emission sources to protect the health of occupants.
Table 9 shows the selected VOC pollutants in the WHO guidelines for indoor air quality [43].
VOC | Possible sources indoors | Possible outcomes | Guidelines |
Benzene (C6H6) | – Cigarette smoke – Burning incense, – new buildings (certain Furnishing and/or polymeric materials) – Cleaning – Painting – Printer – Kerosene stove | – Acute myeloid leukemia – Genotoxicity | – No safe level of exposure can be recommended. – The unit risk for leukemia per 1 μg/m³ air concentration is 6 × 10⁻⁶. – The benzene concentrations in the air associated with an elevated lifetime risk of 1 in 10,000, 1 in 100,000, and 1 in 1,000,000 are 17, 1.7, and 0.17 μg/m³, respectively. |
Formaldehyde (CH2O) | – Combustion processes such as smoking, heating, cooking, or burning candles or incense – Building materials and consumer goods (new materials and products) | Sensory input | 0,1 mg/m3 (30 minute average concentration). |
Naphthalene (C10H8, belongs to PAH) | – Kerosene heaters without ventilation – Cigarette smoke – Naphthalene insect repellents (mothballs) – Solid deodorant blocks for toilets containing naphthalene – Wood smoke, heating oil, and gasoline – Creosote used for wood preservation | Respiratory damage that led to inflammation and malignancy in animal studies. | 0,01 mg/m3 (yearly average concentration). |
Polycyclic Aromatic Hydrocarbons (PAH, CmHn) | – Smoking – Cooking – furnace heating in the household and an open fireplace – Emissions from incense | Lung cancer (B[a]P is one of the strongest carcinogens among known PAHs) | – No threshold value can be established, and all indoor exposures are considered health-relevant. – The unit risk for lung cancer is estimated for PAH mixtures at 8.7 × 10⁻⁵ per ng/m³ of B[a]P. – The corresponding concentrations for lifetime exposure to B[a]P that lead to an elevated lifetime cancer risk of 1 in 10,000, 1 in 100,000, and 1 in 1,000,000 are approximately 1.2, 0.12, and 0.012 ng/m³, respectively. |
Trichlorethylene (TCE, C2HCl3) | – Contaminated drinking water – Consumer products (wood stains, paints, finishes, lubricants, adhesives, correction fluid, paint strippers, and certain cleaning products) – Food | Carcinogenicity (liver, kidney, bile duct and non-Hodgkin’s lymphoma), with the assumption of genotoxicity. | – Risk unit estimate of 4.3 × 10⁻⁷ per μg/m³. – The concentrations of TCE in the air associated with an elevated lifetime cancer risk of 1 in 10,000, 1 in 100,000, and 1 in 1,000,000 are 230, 23, and 2.3 μg/m³, respectively. |
Tetrachlorethylene (PCE, C2Cl4) | – Consumer products (adhesives, fragrances, stain removers, etc.) – Contaminated drinking water | Effects on the kidneys indicating early kidney disease and impaired neurological function. | Carcinogenicity (liver, kidney, bile duct, and non-Hodgkin’s lymphoma), with the assumption of genotoxicity. |
Carbon dioxide CO2
In contrast to chemical pollutants from the group of volatile organic compounds (VOCs), CO2 has been used as an indirect parameter for assessing ventilation. However, CO2 is not included in the list of selected indoor air pollutants in the WHO guidelines [43]. That is due to the fact, that high CO2 concentrations can indicate inadequate ventilation and a buildup of indoor air pollutants [33].
DIN EN 16798-1 [8] provides the procedure for designing ventilation systems based on CO2 concentrations. The recommended threshold values for CO2 concentration in indoor spaces are listed in Table 10.
Category | Design value of the ΔCO2 concentration in living rooms [ppm above the value in outdoor air] | Design value of the ΔCO2 concentration in living rooms (Assumption: CO2 concentration outdoor is 400 ppm) [ppm] |
I | 550 | 950 |
II | 800 | 1200 |
III | 1350 | 1750 |
IV | 1350 | 1750 |
According to the 2008 announcement by the German Federal Office for Environment (Umweltbundesamt) [47], the concentration of CO2 in outdoor air is 400 ppm.
Another widely recognized reference value for CO2 concentration is the 1000 ppm threshold proposed by Pettenkofer in 1858 [48], which has been adopted by several standards and guidelines [47, 49, 51, 52]. Indoor air with less than 1000 ppm of CO2 is considered safe. Table 11 summarizes the reference values for CO2 in indoor air according to various standards and guidelines.
Norm / Guideline | Benchmark for CO2 (absolute value) or ΔCO2-concentration (difference between indoor and outdoor) in ppm |
CEN/EN 16798-1 (based on German version DIN EN 16798-1:2019) [8] | Category I: ΔCO2 ≤ 550 ppm Category II: ΔCO2 ≤ 800 ppm Category III: ΔCO2 ≤ 1350 ppm Category IV: ΔCO2 > 1350 ppm |
The law for enviornmental health in buildings, LEHB [49] | Acceptable: CO2 ≤ 1000 ppm Unacceptable: CO2 > 1000 ppm |
Singapur Standard SS 554:2016 [50] | Acceptable: ΔCO2 ≤ 700 ppm Unacceptable: ΔCO2 > 700 ppm |
Hong Kong Environmental Protection Department [51] | Excellent: CO2 ≤ 800 ppm Good: CO2 ≤ 1000 ppm Unacceptable: CO2 > 1000 ppm |
German Environment Agency (Deutscher Umweltbundesamt) [47] | Hygienically harmless: CO2 ≤ 1000 ppm Hygienically conspicuous: CO2 ≤ 2000 ppm Hygienically unacceptable: CO2 > 2000 ppm |
Department of Occupational Safety and Health (DOSH) Malaysia [52] | Acceptable: CO2 ≤ 1000 ppm Unacceptable: CO2 > 1000 ppm |
In scientific literature and practice, the 1000 ppm threshold is often cited as a reference for good Indoor Air Quality (IAQ), with the ASHRAE Standard 62.1 [53] frequently being mentioned as the source for this value. However, this statement is incorrect. The CO2 guideline value was removed from the ASHRAE standard as early as 1999. This removal was based on the understanding that CO2 is not a direct source of air pollution, but rather an indirect indicator of ventilation [54-56].
The CO2 concentration in indoor spaces does not provide a complete picture of IAQ. It can only be a useful tool for evaluating IAQ if users are aware of the following limitations [55, 56]:
- The number and activity level of occupants can influence CO2 measurements.
- The duration of room occupancy can affect CO2 readings.
- It should be ensured that there are no combustion processes or other CO2 sources that could affect the measurements.
- While CO2 levels below a certain threshold do not guarantee overall acceptable IAQ, CO2 levels significantly above the expected range may indicate that the ventilation system is not functioning properly.
- The accuracy of sensors, their location, and calibration are crucial for drawing meaningful conclusions from measured CO2 concentrations.
- Air purification technologies that only remove CO2 do not necessarily improve overall IAQ and may interfere with systems that use CO2 for ventilation control or IAQ monitoring.
Although some literature sources suggest a correlation between high CO2 concentrations and the well-being and performance of occupants, the causal relationship needs to be further and more thoroughly investigated [57]. On the other hand, outdoor CO2 concentrations are not constant and are influenced by factors such as region and season. At the same time, atmospheric CO2 levels are increasing year by year due to climate change [58-60]. Therefore, when applying the relevant guidelines, the local outdoor CO2 concentration should be taken into account, especially if the guidelines specify absolute CO2 levels rather than concentration differentials.
6. Cross-modal effects
As early as the 1990s, it was recognized that complaints and health effects related to indoor climate were not caused by a single parameter [61]. In a chamber test with 99 young adults, IEQ (Indoor Environmental Quality) factors such as thermal comfort, indoor air quality, and the acoustic and visual environment had a combined effect on the acceptance of the environment and the performance of users [62].
The physical environmental parameters are interconnected, and the perception of comfort is a composite state that includes users’ sensations regarding all of these factors [19]. For instance, relative humidity plays a crucial role not only in thermal comfort, as defined in the PMV/PPD model, but also as a factor influencing indoor air quality. Nagda and Hodgson [63] found in their study that a slight increase in relative humidity positively impacted the perceived indoor air quality. This conclusion was also supported by Wolkoff and Kjærgaard [64]. Additionally, personal factors such as gender, age, health status, duration of occupancy, or work also influence the assessment of indoor climate comfort [23].
The subjective evaluation of comfort in relation to various physical environmental parameters also varies depending on their specific characteristics. The study by Huang et al. [19] demonstrated that thermal and acoustic comfort play a crucial role in assessing overall satisfaction with the indoor climate. This implies that the entire indoor environment is perceived as unacceptable once the temperature or noise level falls outside the acceptable range. Huang et al. [19] also found that occupants can tolerate a wider temperature range if the noise level is low, and a higher noise level is accepted when thermal comfort is high. Additionally, occupants may perceive the indoor environment as acceptable even with dim lighting, as long as thermal and acoustic comfort are high.
The study conducted by Frontczak et al. [23] demonstrated that thermal comfort has a significant influence on overall indoor climate comfort, which aligns with the findings of Huang et al. [19]. Humphreys’ study [65] showed that visual comfort has the least impact on overall indoor climate comfort. Even when lighting intensity falls outside the acceptable range, the indoor environment can still be perceived as acceptable [34].
Chinazzo et al. [66] and Wu et al. [67] summarized these interactions into two categories, as illustrated in Figure 6:
- Cross-modal effect: It occurs when one stimulus influences a response which is usually triggered by a different, unrelated stimulus. For example, light affecting thermal responses.
- Combined effect: This takes place when multiple stimuli together influence a response that isn’t directly linked to a specific indoor stimulus, such as individual perceptions like general well-being, physical condition, behavior, physiology, or performance. These stimuli can come from the environment or other areas like personal surroundings or context.

A classic example of a cross-modal effect is the Hue-Heat Effect, which refers to the relationship between color temperature and thermal perception (cf. [74]). In this effect, a warmer-colored environment is perceived as physically warmer, while a cooler-colored environment feels colder.
To assess or predict indoor climate comfort and satisfaction, numerous multi-domain models have been developed. These models, summarized by Wu et al. [67], are illustrated in Figure 7.

7. Which comfort models are used in the Ressourcenwächter?
See Comfort models behind Ressourcenwächter
Literature
- [1] Fanger, P. O. 1970. Thermal comfort. Copenhagen: Danish Technical Press.
- [2] DIN EN ISO 7730:2005. “Ergonomie der thermischen Umgebung – Analytische Bestimmung und Interpretation der thermischen Behaglichkeit durch Berechnung des PMV und des PPD-Indexes und Kriterien der lokalen thermischen Behaglichkeit.”
- [3] Sarbu, Ioan and Calin Sebarchievici. 2013. “Aspects of indoor environmental quality assessment in buildings.” Energy and Buildings 60:410–419.
- [4] van Hoof, J., M. Mazej and J.L.M. Hensen. 2010. “Thermal comfort: research and practice.” Frontiers in Bioscience 15(2):765–788.
- [5] de Dear, Richard J. and Gail Schiller Brager. 1998. “Developing an Adaptive Model of Thermal Comfort and Preference.” ASHRAE Transactions 104:145–167.
- [6] de Dear, Richard J., Gail Brager and Donna Cooper. 1997. Developing an adaptive model of thermal comfort and preference: final report [on] ASHRAE RP-884. Macquarie Research Limited.
- [7] de Dear, Richard J. 2004. “Thermal comfort in practice.” Indoor Air 14:32–39.
- [8] DIN EN 16798-1:2019. “Energetische Bewertung von Gebäuden – Lüftung von Gebäuden – Teil 1: Eingangsparameter für das Innenraumklima zur Auslegung und Bewertung der Energieeffizienz von Gebäuden bezüglich Raumluftqualität, Temperatur, Licht und Akustik.”
- [9] ANSI/ASHRAE Standard 55-2017: “Thermal Environmental Conditions for Human Occupancy”.
- [10] Yao, R., Li, B., & Liu, J. (2009). A theoretical adaptive model of thermal comfort–Adaptive Predicted Mean Vote (aPMV). Building and Environment, 44(10), 2089-2096.
- [11] Fanger, P. O., & Toftum, J. (2002). Extension of the PMV model to non-air-conditioned buildings in warm climates. Energy and Buildings, 34(6), 533-536.
- [12] Fiala, D. (1998). Dynamic Simulation of Human Heat Transfer and Thermal Comfort, Doctoral dissertation, De Montfort University.
- [13] Zhang, H., Arens, E., Huizenga, C., & Han, T. (2010). Thermal sensation and comfort models for non-uniform and transient environments: Part I: Local sensation of individual body parts. Building and Environment, 45(2), 380–388. https://doi.org/10.1016/j.buildenv.2009.06.018
- [14] Zhang, H., Arens, E., Huizenga, C., & Han, T. (2010). Thermal sensation and comfort models for non-uniform and transient environments, part II: Local comfort of individual body parts. Building and Environment, 45(2), 389–398. https://doi.org/10.1016/j.buildenv.2009.06.015
- [15] Zhang, H., Arens, E., Huizenga, C., & Han, T. (2010). Thermal sensation and comfort models for non-uniform and transient environments, part III: Whole-body sensation and comfort. Building and Environment, 45(2), 399–410. https://doi.org/10.1016/j.buildenv.2009.06.020
- [16] Schmidt, C. (2016). Entwicklung eines Modellansatzes zur Bewertung der thermischen Behaglichkeit unter inhomogenen Klimabedingungen [Development of a model approach for the prediction of human thermal comfort at inhomogenous ambient conditions], Doctoral dissertation, RWTH Aachen University.
- [17] Schweiker, M. (2022). Combining adaptive and heat balance models for thermal sensation prediction: A new approach towards a theory and data‐driven adaptive thermal heat balance model. Indoor Air, 32(3), e13018.
- [18] Arakawa Martins, L., Soebarto, V., & Williamson, T. (2022). A systematic review of personal thermal comfort models. Building and Environment, 207, 108502. https://doi.org/10.1016/j.buildenv.2021.108502
- [19] Huang, Li, Yingxin Zhu, Qin Ouyang and Bin Cao. 2012. “A study on the effects of thermal, luminous, and acoustic environments on indoor environmental comfort in offices.” Building and Environment 49:304–309.
- [20] Zhang, D., Wong, L.-T., Mui, K.-W., & Tang, S.-K. (2024). Acoustic comfort in educational buildings: An integrative review and new directions for future research. Building and Environment, 262, 111849. https://doi.org/10.1016/j.buildenv.2024.111849
- [21] World Health Organization (WHO), Burden of Disease from Environmental Noise Quantification of Healthy Life Years Lost in Europe, 2011. Copenhagen.
- [22] Navai, M. and J.A. Veitch. 2003. “Acoustic Satisfaction in Open-Plan Offices: Review and Recommendations.” Institute for Research in Construction, National Research Council Canada RR-151.
- [23] Frontczak, Monika and Pawel Wargocki. 2011. “Literature survey on how different factors influence human comfort in indoor environments.” Building and Environment 46(4):922–937.
- [24] Cowan, James P. 1994. Handbook of environmental acoustics. Wiley. URL: https://www.wiley.com/en-us/Handbook+of+Environmental+Acousticsp-9780471285847
- [25] Keighley, E.C. 1966. “The determination of acceptability criteria for office noise.” Journal of Sound and Vibration 4(1):73–87.
- [26] Warnock, A.C.C. 1973. “Acoustical privacy in the landscaped office.” The Journal of the Acoustical Society of America 53(6):1535–1543. https://doi.org/10.1121/1.1913498
- [27] Keighley, E.C. and P.H. Parkin. 1979. “Subjective response to sound conditioning in a landscaped office.” Journal of Sound and Vibration 64(3):313–323.
- [28] DIN EN 12464-1:2019: “Licht und Beleuchtung – Beleuchtung von Arbeitsstätten – Teil 1: Arbeitsstätten in Innenräumen.”
- [29] Laborde, Joyce M., William A. Dando and Marjorie J. Powers. 1986. “Influence of weather on osteoarthritics.” Social Science & Medicine 23(6):549–554.
- [30] von Mackensen, Sylvia, Peter Hoeppe, Abdel Maarouf, Pierre Tourigny and Dennis Nowak. 2005. “Prevalence of weather sensitivity in Germany and Canada.” International Journal of Biometeorology 49(3):156–166. https://doi.org/10.1007/s00484-004-0226-2
- [31] McAlindon, Tim, Margaret Formica, Christopher H. Schmid and Jeremiah Fletcher. 2007. “Changes in Barometric Pressure and Ambient Temperature Influence Osteoarthritis Pain.” The American Journal of Medicine 120:429–434.
- [32] Linde, M., L. Edvinsson, K. Manandhar, A. Risal and T. J. Steiner. 2017. “Migraine associated with altitude: results from a population-based study in Nepal.” European Journal of Neurology 24(8):1055–1061.
- [33] Chojer, H., P.T.B.S. Branco, F.G. Martins, M.C.M. Alvim-Ferraz and S.I.V. Sousa. 2020. “Development of low-cost indoor air quality monitoring devices: Recent advancements.” Science of The Total Environment 727:138385.
- [34] Azuma, K., Yanagi, U., Kagi, N. et al. (2020). Environmental factors involved in SARS-CoV-2 transmission: effect and role of indoor environmental quality in the strategy for COVID-19 infection control. Environ Health Prev Med, vol. 25, p. 66. https://doi.org/10.1186/s12199-020-00904-2
- [35] Peng, Z. & Jimenez J. L. (2021). Exhaled CO2 as a COVID-19 Infection Risk Proxy for Different Indoor Environments and Activities. Environmental Science & Technology Letters, vol. 8, no. 5, pp. 392–397. https://doi.org/10.1021/acs.estlett.1c00183
- [36] Dionova, Brainvendra Widi, M.N. Mohammed, S. Al-Zubaidi and Eddy Yusuf. “Environment indoor air quality assessment using fuzzy inference system.” ICT Express 6(3):185–194.
- [37] Cincinelli, Alessandra and Tania Martellini. 2017. “Indoor Air Quality and Health.” International Journal of Environmental Research and Public Health 14(11).
- [38] Wyon, David P. 2004. “The effects of indoor air quality on performance and productivity.” Indoor air 14:92–101.
- [39] EU-Richtlinie 1999/13/EG. “Richtlinie über die Begrenzung von Emissionen flüchtiger organischer Verbindungen, die bei bestimmten Tätigkeiten und in bestimmten Anlagen bei der Verwendung organischer Lösungsmittel entstehen.”
- [40] Alford, Kyle L. and Naresh Kumar. 2021. “Pulmonary Health Effects of Indoor Volatile Organic Compounds—A Meta-Analysis.” International Journal of Environmental Research and Public Health 18(4).
- [41] U.S. Environmental Protection Agency (EPA). Volatile Organic Compounds’ Impact on Indoor Air Quality. Accessed 17. August 2021, from https://www.epa.gov/indoor-air-quality-iaq/volatile-organic-compounds-impact-indoor-air-quality
- [42] Pettit, T., P.J. Irga and F.R. Torpy. 2018. “Functional green wall development for increasing air pollutant phytoremediation: Substrate development with coconut coir and activated carbon.” Journal of Hazardous Materials 360:594–603.
- [43] World Health Organization. (2010). WHO guidelines for indoor air quality: Selected pollutants. WHO.
- [44] U.S. Environmental Protection Agency (EPA) (2006). Guidelines for reporting of daily air quality—Air Quality Index (AQI) (Nummer EPA-454/B-06-001).
- [45] Sensirion AG. Sensirion Gas Index Algorithm. GitHub. Accessed 19. August 2024, from https://github.com/Sensirion/gas-index-algorithm
- [46] Sensirion AG. Datasheet SGP40: Indoor Air Quality Sensor for VOC Measurements. Accessed 19. August 2024, from https://sensirion.com/media/documents/296373BB/6203C5DF/Sensirion_Gas_Sensors_Datasheet_SGP40.pdf
- [47] Umweltbundesamt (2008). Gesundheitliche Bewertung von Kohlendioxid in der Innenraumluft [Health assessment of carbon dioxide in indoor air]. Accessed 19. August 2024, from https://www.umweltbundesamt.de/sites/default/files/medien/pdfs/kohlendioxid_2008.pdf
- [48] Pettenkofer (1858). Besprechung Allgemeiner auf die Ventilation bezüglicher Fragen. Über den Luftwechsel in Wohngebäuden. [Discussion of general questions relating to ventilation. About air exchange in residential buildings]. J.G. Cottaísche Buchhandlung, München
- [49] Ministry of the Environment, Government of Japan. The law for enviornmental health in buildings (LEHB).
- [50] National Environment Agency, Singapore (2016). Singapore standard SS 554:2016 – Code of Practice for Indoor Air Quality for Air-Conditioned Buildings.
- [51] Hong Kong Enviornmental Protection Department (2019). Hongkong Guidance Notes for the Management of Indoor Air Quality in Offices and Public Places.
- [52] Department of Occupational Safety and Health (DOSH) Malaysia (2010). Industry Code of Practice on Indoor Air Quality 2010 (ICOP IAQ 2010).
- [53] ANSI/ASHRAE Standard 62.1-2022: Ventilation and Acceptable Indoor Air Quality.
- [54] Persily, A. (2020). Quit Blaming ASHRAE Standard 62.1 for 1000 ppm CO2. Indoor Air 2020, The 16th Conference of the International Society of Indoor Air Quality & Climate.
- [55] ASHRAE. (2022). ASHRAE Position Document on Indoor Carbon Dioxide.
- [56] ASHRAE. (2023). INDOOR CARBON DIOXIDE, VENTILATION AND INDOOR AIR QUALITY.
- [57] Fisk, W., Wargocki, P., & Zhang, X. (2019). Do Indoor CO2 Levels Directly Affect Perceived Air Quality, Health, or Work Performance? ASHRAE Journal.
- [58] Pawlak, W., Fortuniak, K., Siedlecki, M. (2010). Carbon dioxide flux in the centre of Łódź, Polandanalysis of a 2-year eddy covariance measurement data set. International Journal of Climatology, vol. 31, issue 2, pp. 232-243. https://doi.org/10.1002/joc.2247
- [59] Song, T., Wang, Y. (2012). Carbon dioxide fluxes from an urban area in Beijing. Atmospheric Research, vol. 106, pp. 139-149. https://doi.org/10.1016/j.atmosres.2011.12.001
- [60] Deutscher Wetterdienst (DWD), Atmospheric greenhouse gas measurements: CO2. Accessed 19. August 2024, from https://www.dwd.de/DE/forschung/atmosphaerenbeob/zusammensetzung_atmosphaere/spurengase/inh_nav/klimagase_node.html
- [61] Fransson, Niklas, Daniel Västfjäll and Jennie Skoog. 2007. “In search of the comfortable indoor environment: A comparison of the utility of objective and subjective indicators of indoor comfort.” Building and Environment 42(5):1886–1890.
- [62] Clausen, Geo and David P. Wyon. 2008. “The Combined Effects of Many Different Indoor Environmental Factors on Acceptability and Office Work Performance.” HVAC&R Research 14(1):103–113.
- [63] Nagda, Niren L and Michael Hodgson. 2001. “Low relative humidity and aircraft cabin air quality.” Indoor air 11(3):200–214.
- [64] Wolkoff, Peder and Søren K Kjærgaard. 2007. “The dichotomy of relative humidity on indoor air quality.” Environment international 33(6):850–857.
- [65] Humphreys, Michael A. 2005. “Quantifying occupant comfort: are combined indices of the indoor environment practicable?” Building Research & Information 33(4):317–325. https://doi.org/10.1080/09613210500161950
- [66] Chinazzo, G., Andersen, R. K., Azar, E., Barthelmes, V. M., Becchio, C., Belussi, L., Berger, C., Carlucci, S., Corgnati, S. P., Crosby, S., Danza, L., de Castro, L., Favero, M., Gauthier, S., Hellwig, R. T., Jin, Q., Kim, J., Sarey Khanie, M., Khovalyg, D., … Wei, S. (2022). Quality criteria for multi-domain studies in the indoor environment: Critical review towards research guidelines and recommendations. Building and Environment, 226, 109719. https://doi.org/10.1016/j.buildenv.2022.109719
- [67] Wu, H., Wu, Y., Sun, X., & Liu, J. (2020). Combined effects of acoustic, thermal, and illumination on human perception and performance: A review. Building and Environment, 169, 106593. https://doi.org/10.1016/j.buildenv.2019.106593
- [68] B. Cao, Q. Ouyang, Y. Zhu, et al., Development of a multivariate regression model for overall satisfaction in public buildings based on field studies in Beijing and Shanghai, Build. Environ. 47 (2012) 394–399.
- [69] L.T. Wong, K.W. Mui, P.S. Hui, A multivariate-logistic model for acceptance of indoor environmental quality (IEQ) in offices, Build. Environ. 43 (1) (2008) 1–6.
- [70] M. Ncube, S. Riffat, Developing an indoor environment quality tool for assessment of mechanically ventilated office buildings in the UK–A preliminary study, Build. Environ. 53 (2012) 26–33.
- [71] F. Fassio, A. Fanchiotti, R. Vollaro, Linear, non-linear and alternative algorithms in the correlation of IEQ factors with global comfort: a case study, Sustainability 6 (11) (2014) 8113–8127.
- [72] A.C.K. Lai, K.W. Mui, L.T. Wong, et al., An evaluation model for indoor environmental quality (IEQ) acceptance in residential buildings, Energy Build. 41 (9) (2009) 930–936.
- [73] T. Guo, S. Hu, G. Liu, Evaluation model of specific indoor environment overall comfort based on effective-function method, Energies 10 (10) (2017) 1634.
- [74] Toftum, J., Thorseth, A., Markvart, J., Logadóttir, Á. (2018). „Occupant response to different correlated colour temperatures of white LED lighting“ (PDF). Building and Environment. 143: 258–268.
- [75] Hu, Z., Wu, J., Yang, L., Gu, Y., & Ren, H. (2021). Physiological and perceptual responses of exposure to different altitudes in extremely cold environment. Energy and Buildings, 242, 110844. https://doi.org/10.1016/j.enbuild.2021.110844
- [76] Liu, X., Zhang, H., Liu, S., Ye, T., Na, Y., Tu, Z., & Zheng, W. (2024). Impacts of barometric pressure on metabolic rate: A plateau experimental study. Energy and Buildings, 321, 114640. https://doi.org/10.1016/j.enbuild.2024.114640
- [77] Moraga, F. A., Osorio, J., Jiménez, D., Calderón-Jofré, R., & Moraga, D. (2019). Aerobic Capacity, Lactate Concentration, and Work Assessment During Maximum Exercise at Sea Level and High Altitude in Miners Exposed to Chronic Intermittent Hypobaric Hypoxia (3,800 m). Frontiers in Physiology, 10, 1149. https://doi.org/10.3389/fphys.2019.01149
- [78] Ohno, H., Kuno, S., Saito, T., Kida, M., & Nakahara, N. (1991). The effects of hypobaric conditions on man’s thermal responses. Energy and Buildings, 16(1–2), 755–763. https://doi.org/10.1016/0378-7788(91)90048-8
- [79] Ruivo, C. R., Da Silva, M. G., & Broday, E. E. (2021). Methodology for calculating an atmospheric pressure-sensitive thermal comfort index PMVaps. Energy and Buildings, 240, 110887. https://doi.org/10.1016/j.enbuild.2021.110887
- [80] Tanabe, S., Kobayashi, K., Nakano, J., Ozeki, Y., & Konishi, M. (2002). Evaluation of thermal comfort using combined multi-node thermoregulation (65MN) and radiation models and computational ¯uid dynamics (CFD). Energy and Buildings.
- [81] Kobayashi, Y., & Tanabe, S. (2013). Development of JOS-2 human thermoregulation model with detailed vascular system. Building and Environment, 66, 1–10. https://doi.org/10.1016/j.buildenv.2013.04.013
- [82] Takahashi, Y., Nomoto, A., Yoda, S., Hisayama, R., Ogata, M., Ozeki, Y., & Tanabe, S. (2021). Thermoregulation model JOS-3 with new open source code. Energy and Buildings, 231, 110575. https://doi.org/10.1016/j.enbuild.2020.110575